Poster for OMNI Internship @ Oak Ridge National Laboratory, 2024
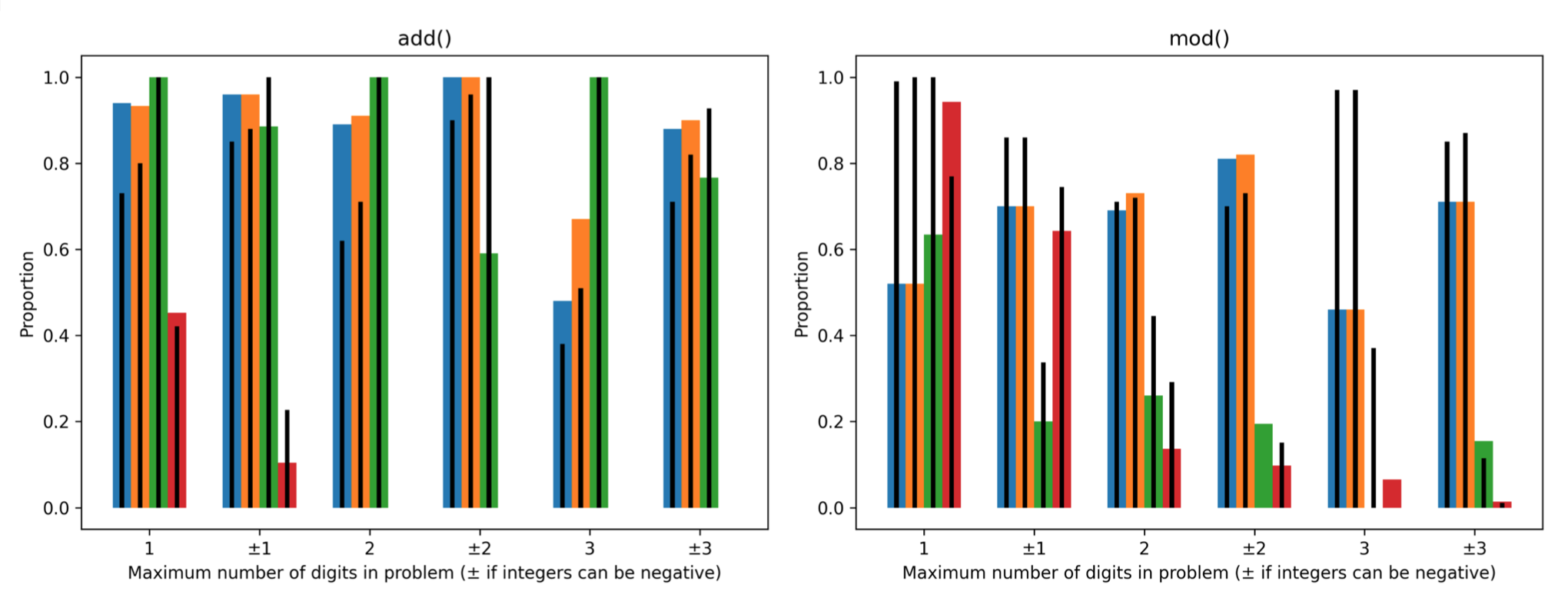
Quick Links
Media
- Self-Hosted Publication
© Thomas Randall 2024. This is the author’s version of the work. It is posted here for your personal use. Not for redistribution.
Publication Details
Appears in DOE Cybersecurity and Technology Innovation 2024 by authors: Thomas Randall, Rong Ge, and Prasanna Balaprakash
Abstract
Large Language Models (LLMs) capture a certain amount of world knowledge spanning many general and technical topics, including programming and performance. Without fine-tuning, the use of In-Context Learning (ICL) can specialize LLM outputs to perform complex tasks. In this work, we seek to demonstrate the regressive capabilities of LLMs in a performance modeling capacity. We find initial evidence that may limit LLM utility even after fine-tuning.